As CES closes its doors, there’s no doubt that the prize for innovation goes to the Rabbit R1. This clever little rabbit, which fits in your pocket, was presented to us in a keynote worthy of the iPhone unveiling, and confirms our 2023 predictions about the multiplication of assistants boosted by generative AI (ChatGPT, Bard, etc.).
The Rabbit R1 is a virtual assistant that learns to handle your digital tasks. Just hit a button, give a voice command, and let the automated scripts (called “rabbits”) do the work for you.
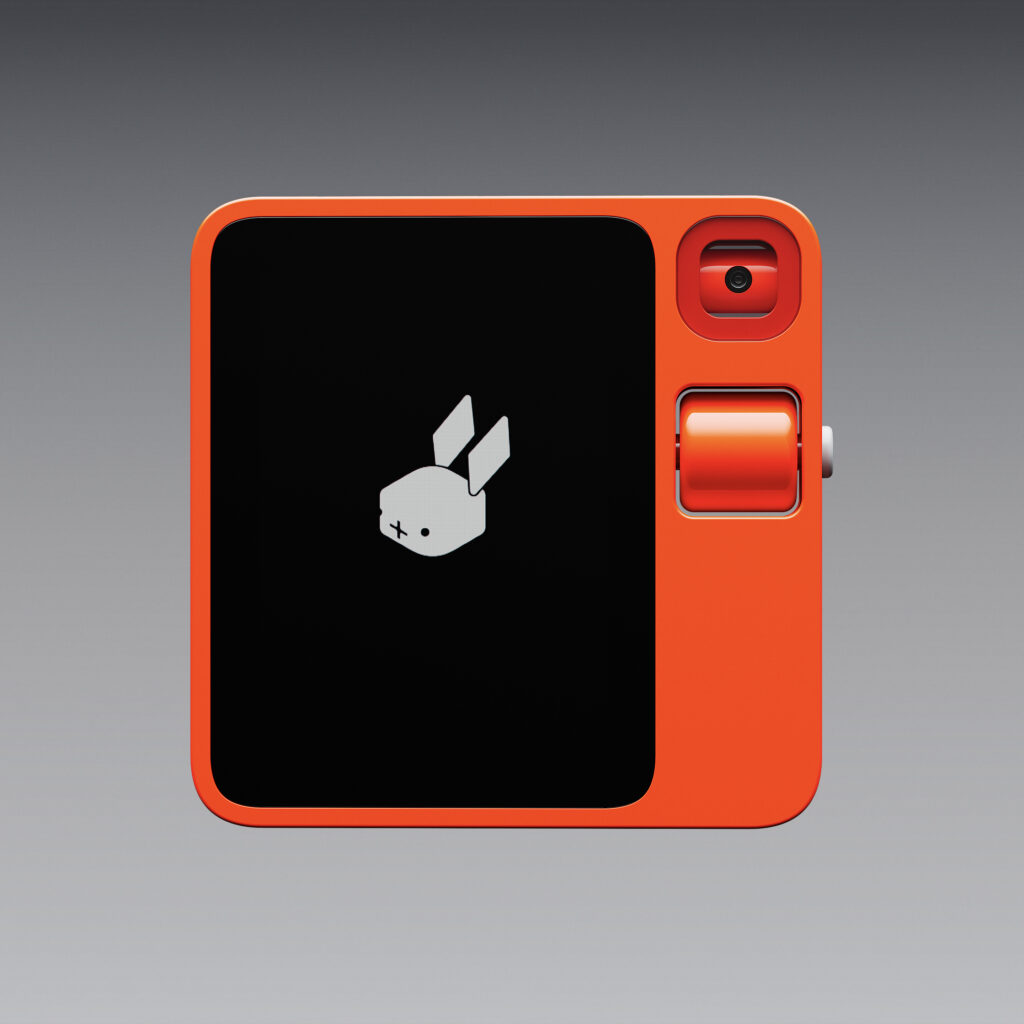
But what I find particularly astonishing is not that this little animal feeds on LLM-based generative AI. That was expected, it’s just happening a little earlier than we thought it would. No, it’s the interconnection of these LLMs with the underlying services that really makes a difference.
The real revolution of Rabbit R1
Indeed, we all imagined that, in a very conventional way, these LLMs would connect to major Internet services via AI-oriented business APIs. This remains the target model for an ecosystem in which generative AI takes center stage, consuming and composing the various services (route calculation, train reservations, account access, etc.).
But the major problem with this target model is that enterprises are lagging in terms of exposing and making these APIs available.
To make up for this delay and still offer a quality service, the Santa Monica startup, parent company of RabbitR1, which has already pre-sold over 10,000 Rabbit R1s during CES (whereas forecasts delivered to analysts were based on 500), is therefore going to supplement this LLM with a LAM, a “Large Action Model.”
This LAM is the Rabbit’s key ingredient.
LAM vs LLM – understanding the difference
Unlike LLMs, which create intelligence through data stores and service access APIs, LAMs are capable of reproducing human activity on a conventional interface (WEB interface, application interface).
This chart, inspired by the one shared by SuperAGI on X, illustrates in greater detail the distinctions between LAM and LLM:
Aspects | LLMS | LAMS |
Large Language Models | Large Agentic(or Action) Models | |
Core Function | Language understanding and generation | Language understanding, generation, complex reasoning and actions |
Primary Strength | Formal linguistic capabilities, generating coherent and contextually relevant text | Advanced linguistic capabilities / (Formal + Functional) combined with multi-hop thinking and generating actionable outputs |
Reasoning Ability | Limited to single-step reasoning based on language patterns | Advanced multi-step reasoning, capable of handling complex, interconnected tasks & goals |
Contextual Understanding | Good at understanding context within text, but limited in applying external knowledge | Superior in understanding and applying both textual and external context |
Problem-Solving | Can provide information and answer questions based on existing data | Can propose solutions, strategic planning, make reasoned decisions and act autonomously |
Learning Approach | Primarily based on pattern recognition from large datasets | Integrates pattern recognition, self-assessment & learning with advanced learning algorithms for reasoning and decision-making |
Application Scope | Suitable for tasks like content creation, simple Q&A, translations, chatbots, etc. | Suitable for building autonomous applications that requires strategic planning, advanced research, and specialized task execution |
Towards AGI | A step in the journey towards Artificial General Intelligence, but with limitations | Represents a significant leap towards achieving Artificial General Intelligence |
Somewhat akin to the screen scraping tools of yesteryear, the owners of the Rabbit R1 must entrust it with their digital identities (bank access code, etc.) so that the LAMs can connect to the bank’s website to consult account balances and suggest only travel offers that fit within their budget, for example.
This new way of accessing services should challenge us on several levels, because if this costly AI technology (i.e. GPU) is necessary, it’s because:
- Service providers have not been quick enough to open up their core systems’ business APIs
- The few services that are exposed are not exposed in such a way as to be easily discovered and consumed by AI models
Could it be that these LAM technologies are turning academic discourse on integration on its head, and offering a bonus to latecomers and poor performers who haven’t correctly APIfied their information systems?
Is this a return to the archaic screen-based integration so derided by purists? Wouldn’t this method used by LAMs be sufficient to open up services?
This would a misunderstanding of how these tools work.
APIfication is more urgent than ever
LAMs are not initially designed to compensate for the absence of service access APIs. They are designed to break down processes into actionable steps.
The cost of using these tools to access simple information such as accounts services is far more expensive and complex than a simple API call.
The result will be a kind of competition for access to services, with the simplest being prioritized by generative AIs, which will go for the shortest route to a faster response. And services that are well APIfied and well exposed via an AI-driven marketplace will undeniably be more successful than those that require heavy CPU minutes to activate.
The APIfication of information systems must therefore be CIOs’ top priority in the next few years. An APIfication that is open to human developers and, of course, to AIs.
At Axway, we help companies by providing the solutions that enable them to carry out this APIfication in complete security and with full governance, but we can also support you in the design and implementation of your digital strategy.
Learn how to unify all your API assets so they’re easier to consume.